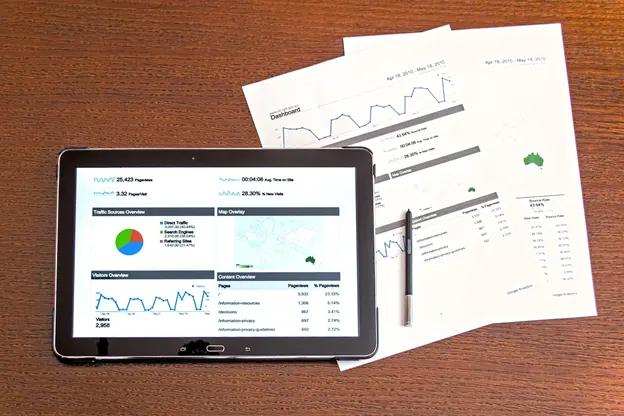
Innovators developing artificial intelligence (AI) solutions to enhance operations, goods, and services across a range of industries make up one side of the AI world. The other side of this innovative sector consists of startups improving AI features to ensure faster, more accurate, and more diverse applications of AI. Cerebras Systems is one such unicorn, offering solutions for vast data processing.
Hence, this article looks at this unicorn's solutions and the disruption it's spearheading in the AI industry.
How Cerebras Systems is Changing Our View of AI Hardware
Cerebras Systems is an attractive investment for individuals interested in the AI hardware sector, given that more companies are leveraging such solutions to reduce the limitations of traditional hardware. This ambitious startup recently filed for an initial public offering, which shows its desire for a considerable stake in the AI hardware sector.
Further, this startup improves its hardware continually, an indicator of its ability to adapt to changing customer needs. For instance, it has three integrated AI processing systems, the latest being the CS-3, encompassing water-scale engine technology.
So, why is Cerebras Systems a market leader?
Wafer-scale Engine
One of the areas this company leads is graphics processing units (GPUs). It's facing out the traditional units that have been popular in AI computational tasks for years by bringing one large chip that can perform the same task. Its engineering makes it different from traditional processors because it's one chip on a single silicon wafer.
On top of that, the initial task of traditional GPUs was rendering graphics, including videos, pictures, and animation. Their capacity to handle parallel processing allows them to work on vast data in real-time, unlike central processing units that perform sequential processing. As such, GPUs can handle non-graphic activities like running complex mathematical models, which makes them a suitable solution for the AI age.
Nevertheless, modern AI training needs have outdone the usefulness of traditional GPUs because companies have to link several of them in a data center. The whole setup of interconnected GPUs requires an efficient cooling system because of the heat it generates, which leads to higher energy costs. A company may require an entire data center for the GPU clusters needed. Further, scaling such systems is expensive and non-eco friendly.
Unlike this traditional setup, the wafer-scale engine (WSE) processes trillions of calculations every second. As such, it's now possible to train AI models faster and more efficiently than the previous GPUs. Further, WSE is the world's largest chip, and Cerebras Systems is now providing the third generation of it, an indication of the popularity and growth of this AI solution.
Energy Efficiency
Traditional GPUs can perform many tasks, but their large-scale computations have a substantial operational cost because they need a high power input. Hence, energy efficiency is a significant contribution from Cerebras Systems since AI model training can be an energy-extensive process.
For instance, using a single chip eliminates communication across several small chips. Therefore, it lowers energy consumption because there is less data movement. You also don't require advanced cooling systems like when using traditional GPU clusters.
Further, this unicorn's focus on energy efficiency is also suitable for the growing trend toward sustainable computing. Companies and research institutions are adapting to the demand to reduce their carbon footprint, which includes adapting their AI systems.
As such, the solutions from Cerebras Systems allow such companies to pursue ambitious artificial intelligence projects while controlling their processing power to reduce environmental impact. A single chip eliminates the need for an energy-consuming data center.
Lower Data Latency
Data latency is one of the constrictions of AI hardware. Traditional GPU clusters have multiple chips that form an intricate network. As such, data travels between these chips during computation, and the external storage is off-chip. This entire system delays data movement and processing, and this problem increases depending on the AI model's complexity and data size.
Using WSE minimizes this problem by bringing in one chip with numerous cores to shorten the distance your data travels. On top of that, WSE reduces the latency further using static random-access memory, which enables on-chip data storage and access.
Scaling Solutions to Meet Diverse Needs
This unicorn positioned itself to cater to companies in different sectors and industries. Hence, it meets the computational needs of companies in diverse sectors, from academia to healthcare and finance.
For instance, the healthcare industry has a myriad of fast data-processing needs. It uses machine-learning algorithms during drug discovery to analyze large datasets to look for patterns, predict effective compounds, or perform other activities needed to accelerate new drug development.
Similarly, companies in the financial sector are embracing AI in risk management. It's a crucial task that involves analyzing vast data, including transaction history, geopolitical activities, and trends in the marketplace. Such predictive modeling allows these institutions to analyze the risk likely to impact investments, trading, and other activities. It doesn't mean traditional GPUs can't handle such data. They can. However, the sheer complexity and volume can overwhelm them.
Thus, this innovative hardware from Cerebras Systems can handle such large datasets and produce accurate insights suitable for financial decisions. The secret lies in the speed because it allows these institutions to utilize assessment strategies like simulation and predictive analysis. These are the tactics behind some trading activities, such as algorithmic trading.
Further, these systems are modular. It allows companies to integrate more WSEs without a significant architectural overhaul. They can increase computing power when necessary without affecting system efficiency.
Conclusion
Cerebras Systems has several solutions to meet the demands of the rapidly growing AI world. For instance, its advanced water-scale engine enables companies to process data faster, which reduces the time they take to train AI models and lowers computational costs.
When you combine the advanced architecture of WSE with the bespoke software from Cerebras Systems, you get a system that handles complex AI workloads more efficiently than traditional systems. This unicorn optimized the processing capability of AI models by building software tailored to the WSE, which enables companies to adjust neural networks based on the system's demands.